pythonで1からニューラルネットワークを実装する第三弾です。今回は、勾配法でパラメーターを更新する為の関数を書きます。いきなり全部のデータでパラメーターを学習すると過学習1
しやすいので、データからランダムにデータを取り出して学習を行うミニバッチ方式を採用します。
前回の記事までで作ったクラスや関数の知識があると仮定しているので、まだの方は以下の記事をどうぞ。
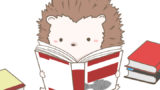
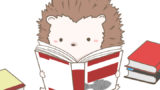
記事で使っているソースコードはgithub に置いてあります。
https://github.com/msamunetogetoge
ミニバッチ方式の実装
ミニバッチ方式は、パラメーターの更新の回数と、1バッチに含まれるデータの数を決める必要があります。今回は適当に更新回数は10000, バッチサイズを100にします。ランダムにデータを選ぶのはnumpy のrandom.choice を使えばできます。コードを書きます。
train_size = train_images.shape[0]
batch_size = 100
iter_mum = 10000
for i in np.arange(iter_mum):
batch_mask = np.random.choice(train_size , batch_size) #1バッチ分のデータ選択
x_batch = train_images[batch_mask]
t_batch = train_labels[batch_mask]
#やらせたいこと
パラメーターの更新の実装
ニューラルネットワークモデルの学習の流れは以下のようになります。
ミニバッチ方式でランダムにデータを取り、勾配を計算し、それぞれのパラメーターを更新しますこれを10000回繰り返し、誤差関数を小さくします。 今回は、100回パラメーターを更新するたびに誤差関数の値と正解率 を記録して、グラフを描いてみます。 このひとまとまりの操作をエポック 2 と言います。 python 3.7 で動くのを確認しているコードを載せます。➁までのコードを持っている人は、以下の事をすれば同じコードになります。
- TwoLayerNet クラスに loss 関数を書き加える
- test_labels をone hot encording する
- パラメーターを更新させるコードをコピペする
import tensorflow as tf
from tensorflow import keras
import numpy as np
import matplotlib.pyplot as plt
from sklearn.preprocessing import OneHotEncoder
import seaborn as sns
sns.set()
#ここまで必要なライブラリの準備
def relu(x):
return np.maximum(0, x)
def relu_grad(x):
grad = np.zeros(x.size).reshape(x.shape)
grad[x>=0] = 1
return grad
def softmax(x):
if x.ndim == 2:
x = x.T
x = x - np.max(x, axis=0)
y = np.exp(x) / np.sum(np.exp(x), axis=0)
return y.T
x = x - np.max(x) # オーバーフロー対策
return np.exp(x) / np.sum(np.exp(x))
def cross_entropy_error(y, t):
if y.ndim == 1:
t = t.reshape(1, t.size)
y = y.reshape(1, y.size)
# 教師データがone-hot-vectorの場合、正解ラベルのインデックスに変換
if t.size == y.size:
t = t.argmax(axis=1)
return -np.sum(np.log(y[np.arange(y.shape[0]), t] + 1e-7)) / y.shape[0]
#ここまで計算が必要な関数の準備
fashion_mnist = keras.datasets.fashion_mnist
(train_images, train_labels), (test_images, test_labels) = fashion_mnist.load_data()
train_images = train_images / 255.0
test_images = test_images / 255.0
train_images = train_images.flatten().reshape(-1,784)
test_images = test_images.flatten().reshape(-1,784)
ohe = OneHotEncoder( sparse=False)
train_labels = ohe.fit_transform(train_labels.reshape(-1,1))
test_labels = ohe.fit_transform(test_labels.reshape(-1,1))
#ここまでデータの整形
#TwoLayerNet クラスの作成
class TwoLayerNet:
def __init__(self, input_size, hidden_size, output_size, weight_init_std=0.01):
self.params = {}
self.params['W1'] = weight_init_std * np.random.randn(input_size, hidden_size)
self.params['b1'] = np.zeros(hidden_size)
self.params['W2'] = weight_init_std * np.random.randn(hidden_size, output_size)
self.params['b2'] = np.zeros(output_size)
def predict(self, x):
W1, W2 = self.params['W1'], self.params['W2']
b1, b2 = self.params['b1'], self.params['b2']
a1 = np.dot(x, W1) + b1
z1 = relu(a1)
a2 = np.dot(z1, W2) + b2
y = softmax(a2)
return y
def accuracy(self, x, t):
y = self.predict(x)
y = np.argmax(y, axis=1)
t = np.argmax(t, axis=1) #tをone hot encording からラベルに戻す
accuracy = np.sum(y == t) / float(x.shape[0])
return accuracy
def gradient(self, x, t):
W1, W2 = self.params['W1'], self.params['W2']
b1, b2 = self.params['b1'], self.params['b2']
grads = {}
# 予測値の計算 (predict と同じ )
a1 = np.dot(x, W1) + b1
z1 = relu(a1)
a2 = np.dot(z1, W2) +b2
y = softmax(a2)
# 各ノードでの微分の計算。出力側から順々に計算する
batch_num = y.shape[0]
dy = (y - t )/ batch_num
grads['W2'] = np.dot(z1.T, dy)
grads['b2'] = np.sum(dy,axis=0)
dz1 = np.dot(dy, W2.T)
da1 = relu_grad(a1) * dz1
grads['W1'] = np.dot(x.T, da1)
grads['b1'] = np.sum(da1,axis=0)
return grads
def loss(self, x, t):
y = self.predict(x)
return cross_entropy_error(y,t)
#パラメーターの更新をさせるコード
network =TwoLayerNet(input_size=784, hidden_size=64 , output_size=10)#隠れ層のノードが64個の、pデリ作成
train_size = train_images.shape[0]
batch_size = 100 #100個のデータを1回の学習に使う
iter_num = 5000 #5000回パラメーターの更新を行う
learning_rate =0.1 #勾配法の定数
loss_list = []
train_acc_list = []
test_acc_list = []
grad_list = []
iter_per_epoch = 100 #100回パラメーターの更新=1エポック
for i in np.arange(iter_num):
batch_mask = np.random.choice(train_size , batch_size) #bastch_size個学習に使うデータを取得する
x_batch = train_images[batch_mask]
t_batch = train_labels[batch_mask]
grad = network.gradient(x_batch, t_batch)
for key in ('W1', 'b1', 'W2', 'b2'):
network.params[key] -= learning_rate * grad[key] #勾配法でパラメーターの更新
if i % iter_per_epoch == 0: #1エポック毎に正答率等を記録させる
grad_list.append(np.linalg.norm(grad["b1"]) ) #パラメーターの更新が止まってないか後で確認したいので記録
loss = network.loss(x_batch, t_batch)
loss_list.append(loss)
train_acc = network.accuracy(train_images, train_labels)
test_acc = network.accuracy(test_images, test_labels)
train_acc_list.append(train_acc)
test_acc_list.append(test_acc)
print("train acc, test acc | " + str(train_acc) + ", " + str(test_acc))
print("Finish Caluculation")
#グラフを描くコード
x = np.arange(len(train_acc_list))
plt.figure(figsize=(13,3))
plt.subplot(1,3,1)
plt.plot(x, train_acc_list, label='Train Acc')
plt.plot(x, test_acc_list, label='Test Acc', linestyle='--')
plt.xlabel("epochs")
plt.ylabel("accuracy")
plt.ylim(0, 1.0)
plt.legend(loc='lower right')
plt.title("Train Acc VS Test Acc")
plt.subplot(1,3,2)
plt.plot(x, loss_list, label=" Error")
plt.xlabel("epochs")
plt.legend()
plt.title("Value Of Loss Function")
plt.subplot(1,3,3)
x = np.arange(len(grad_list))
plt.plot(x, grad_list, label='Norm Of Gradient ')
plt.xlabel("epochs")
plt.legend()
plt.title("Training Of Parameter (b1)")
plt.savefig("train_neuralnet.png")
このコードで学習を実行すると、最終的には正答率87%程度まで到達します。適当に作った行列では5%とかだったので、かなり進歩 3 しました。
また、最後のグラフを描く部分のコードから以下のようなグラフが得られます。
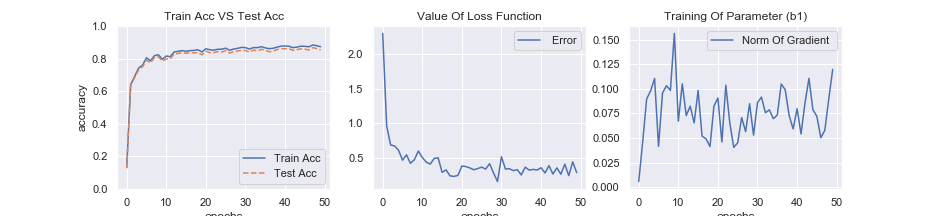
注目すべき点を解説します。
正答率と誤差関数のグラフが連動している事に注目しましょう。正答率が上がる=誤差関数の値が小さくなるという状態になっています。この状態は、モデルとデータに対して適切な誤差関数を選べていることを示しています。
また、学習用に使うデータと、テスト用に使うデータの正答率が殆ど変わらないという事にも注目すべきです。これは、使用したデータが”良い”データだった事を示しています。良い、というのは以下のような意味合いです。
- 学習用データが充分な数存在している
- 学習用データとテスト用データで極端な違いが無い
- 学習に意味のあるデータが沢山ある
モデルが素晴らしかったという可能性ももちろんあります。
最後に、勾配のグラフを見ましょう。最初から最後まで勾配の大きさが変化しています。しかし、10エポック辺りから誤差関数値も正答率も殆ど変化が無いので与えた情報やパラメーターでは既にモデルの学習の限界が来ていると見た方が良いでしょう。
ニューラルネットワークモデルを改良するには層を増やしてみる(層を深くする)、というのがあります。次回からはディープラーニングの実装に挑戦しましょう。
まとめ
・ニューラルネットワークのモデルを1から実装した
・mnist はデータが凄く良い
・次回からはディープラーニングの実装